Researchers propose “UW Method” for identifying observation stays in Medicare Data



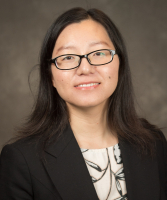
Observation status - in which hospitalized Medicare beneficiaries are classified as outpatients for billing status – posed an intractable problem for health services and care research. Despite observation stay policies being in place for many years, there wasn’t a standard method that health services researchers could rely on to identify observation stay hospitalizations when working with Medicare data sets.
This is a problem, explains Ann Sheehy, MD, MS (pictured above and at top right), associate professor and head, Hospital Medicine. “It's important to have at least one reproducible method so that results can be compared across studies,” she said. Thus, Dr. Sheehy collaborated with Amy Kind, MD, PhD (pictured at middle right), associate professor, Geriatrics and Gerontology and analyst Fangfang Shi, MAS (pictured at lower right), to develop and publish what the team is referring to as the “University of Wisconsin Method."
Medicare observation policies attempt to address the reality of hospitalized patients who are deemed too sick to go home, but not sick enough to be admitted as inpatients. Whether a hospitalization is classified as an inpatient or observation stay has financial implications for Medicare beneficiaries. Medicare patients hospitalized as inpatients are covered by Medicare Part A hospital insurance, and Medicare pays for post-discharge skilled nursing care. Medicare patients hospitalized under observation are covered by Part B, and receive no coverage benefit for discharge to a skilled nursing facility. They also do not receive coverage for any drugs that are self-administered, and hospitals caring for them during their observation stay are reimbursed at a lower rate.
Sleuthing out which hospitalization events were categorized as observation stays in Medicare data sets is not straightforward, however, because classification can change over time. Within health records, medical care is described in alphanumerical codes, and analyzing patterns of codes can suggest - but does not always conclusively confirm - whether a stay was ultimately classified as inpatient or observation. Previous studies in the field of observation stay research did not always define codes or methodology in full, making comparisons impossible. To further complicate matters, stay determination can change; an inpatient admission order may later be categorized as falling under criteria for observation stay, and status can also change upon review or even as a result of appeals processes.
Final categorization of a stay does not eliminate the “residue” of medical codes that persists in the records. “There was no standard research methodology for identifying observation stays exists, including methods to identify and properly characterize ‘status change’ events, which are hospital stays where initial and final inpatient or outpatient (observation) statuses differ,” said Dr. Sheehy.
The researchers analyzed a 2014 national 20 percent random sample of Part A and B Medicare data, which comprised 1.67M hospitalization events. From there, they looked for the presence of specific observation revenue center (ORC) codes identified by Research Data Assistance Center (ResDAC) that corresponded to different types of treatment or observation occurring within 30 days of an inpatient stay. They also looked for presence of defined Healthcare Common Procedure Coding System (HCPCS) codes relating to hospital observation services.
"We quantified how frequently these codes occurred singly or in combination, and also defined coding patterns that signal status changes from outpatient (observation) to inpatient," said Dr. Sheehy.
Of the four potential observation codes identified by ResDAC, the UW team wanted to define which ones represented true observation center revenue codes, and which ones were observation stays that had converted to an inpatient stay down the road. “We started digging into this and were surprised at the mess we found – there were so many outpatient codes associated with inpatient claims,” said Dr. Sheehy. “We were trying to sort out what are the false indicators and what were the real indicators. Observation codes doesn't necessarily end up being billed as an observation stay.”
The total number of observation stays that Dr. Sheehy, Dr. Kind, and Shi identified using their method was approximately equivalent to those identified by two other publications, giving them confidence that the UW Method was accurately identifying observation stays. It held much more value than previous methods for identifying status changes, though. “We found a 47 percent rate of status change from inpatient to observation or vice versa,” said Dr. Sheehy. “This demonstrates ongoing difficulty and confusion with assignment of observation when patients are admitted.”
Having a defined method to identify observations is a critical tool needed for policy refinement. “Researchers can always disagree on whether this is the best method or not, but we felt it was important to be transparent about what codes were present,” said Dr. Sheehy. A common method will help compare apples to apples when analyzing data trends.
“If others would want to modify our definition, they would at least know what codes and methods to use, and could defend and define their own variations on the method.”
The study was supported by the Health Services and Care Research Program at the University of Wisconsin-Madison Department of Medicine.
Resources:
- Sheehy AM, Shi F, Kind AJH. 2018. Identifying Observation Stays In Medicare Data: Policy Implications of a Definition. J Hosp Med. 29:E1-E5.
- "Hospitalists advise changes to regulatory landscape for observation status," Department of Medicine, November 30, 2017
- "Poor medicare patients may spend more on hospital stays," Department of Medicine, October 18, 2017
- "Counting midnights: Medicare and skilled nursing facility benefits," Department of Medicine, March 9, 2017
Photo (top): A study by Dr. Ann Sheehy, above, has established a new method for identifying observation stays in Medicaid data sets. Dr. Sheehy collaborated with programmer analyst Fangfang Shi and Dr. Amy Kind. Photo credit: Clint Thayer/Department of Medicine